Predictive Maintenance Services The Best 1 Ultimate Guide
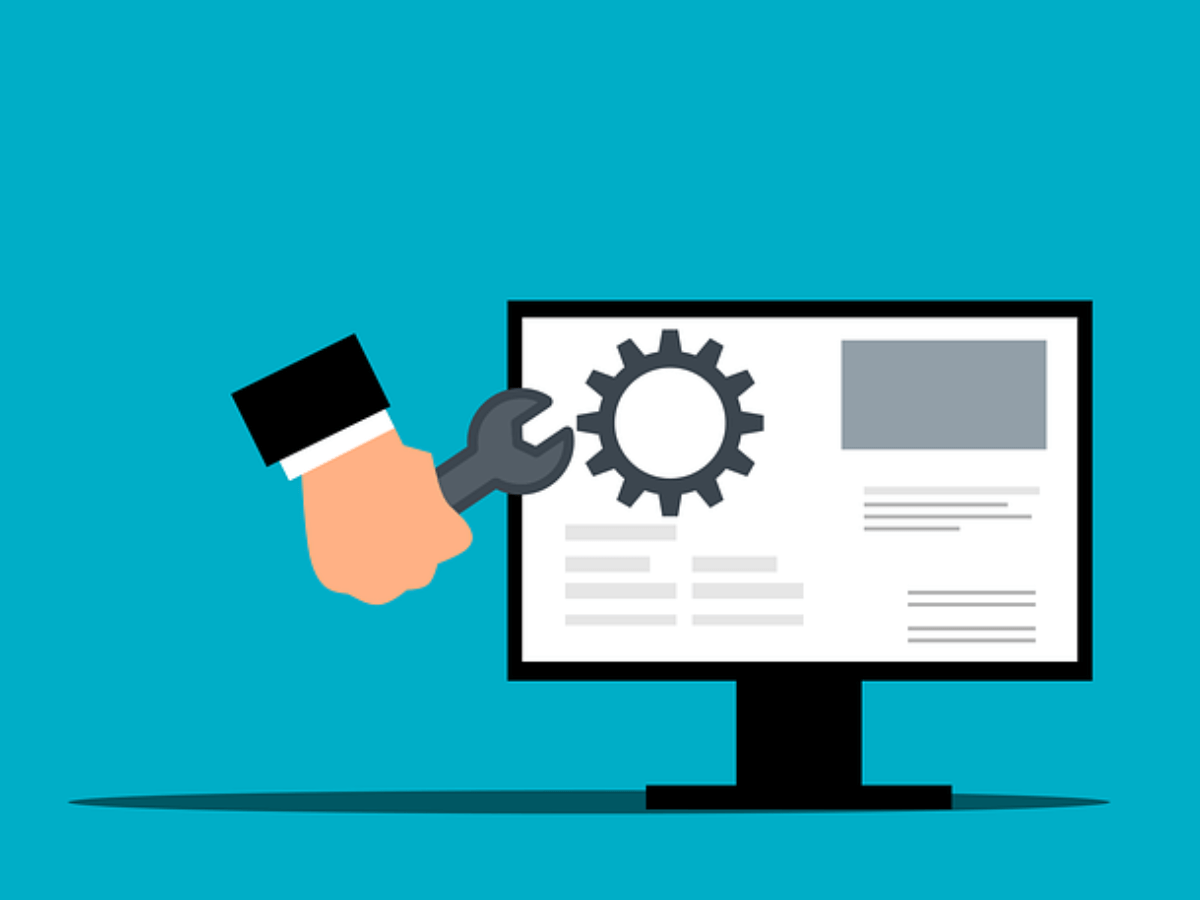
In today’s fast-paced industrial environment, maintaining equipment and machinery is crucial for operational efficiency. Predictive maintenance services have emerged as a game-changer, offering a proactive approach to equipment management. This ultimate guide delves into the world of predictive maintenance services, exploring their benefits, techniques, and how they can transform your operations.
Predictive maintenance services are advanced strategies designed to monitor the condition of equipment and predict when maintenance should be performed. Unlike traditional maintenance methods, which are either reactive or scheduled at fixed intervals, predictive maintenance focuses on predicting equipment failures before they occur. This approach minimizes downtime, reduces repair costs, and enhances the lifespan of machinery.
Reduced Downtime: By predicting potential failures before they happen, predictive maintenance services help prevent unexpected equipment breakdowns. This reduces downtime and ensures that operations continue smoothly.
Cost Savings: Predictive maintenance services can significantly lower maintenance costs. By addressing issues before they become severe, you avoid costly repairs and replacements, leading to long-term savings.
Extended Equipment Lifespan: Regular monitoring and timely interventions extend the life of your equipment. Predictive maintenance services help maintain machinery in optimal condition, preventing premature wear and tear.
Improved Safety: Predictive maintenance services enhance safety by identifying potential problems early. This proactive approach helps prevent accidents and ensures a safer working environment for employees.
Enhanced Efficiency: With predictive maintenance, equipment operates at peak performance. This leads to more efficient processes and better overall productivity.
Predictive maintenance services utilize various techniques and technologies to monitor equipment health. Here’s an overview of the key components involved:
Data Collection: Sensors and IoT devices collect data on equipment performance, including temperature, vibration, and other relevant parameters. This data is crucial for analyzing the health of machinery.
Data Analysis: Advanced analytics and machine learning algorithms analyze the collected data to identify patterns and predict potential failures. This analysis helps determine the optimal time for maintenance.
Condition Monitoring: Continuous monitoring of equipment condition provides real-time insights into performance and wear. Predictive maintenance services use this information to forecast when maintenance is needed.
Maintenance Planning: Based on the predictions, maintenance tasks are scheduled and planned. This ensures that interventions are made at the right time, minimizing disruptions to operations.
To successfully implement predictive maintenance services, consider the following steps:
Assess Your Needs: Evaluate your equipment and identify which machines would benefit most from predictive maintenance services. Prioritize high-value or critical assets for initial implementation.
Choose the Right Tools: Select the appropriate sensors, monitoring systems, and analytics tools that align with your equipment and operational needs.
Train Your Team: Ensure that your maintenance team is trained to use predictive maintenance tools and interpret the data effectively. Proper training is essential for maximizing the benefits of these services.
Monitor and Adjust: Continuously monitor the performance of your predictive maintenance system and make adjustments as needed. Regularly review the data and predictions to refine your maintenance strategies.
Evaluate ROI: Assess the return on investment (ROI) of your predictive maintenance services by tracking improvements in equipment performance, cost savings, and overall efficiency.
Selecting a reputable provider for predictive maintenance services is crucial. Look for a company with a proven track record, advanced technology, and expertise in your industry. Evaluate their offerings and ensure they provide comprehensive support and training.
Predictive maintenance services represent a revolutionary approach to equipment management, offering numerous benefits such as reduced downtime, cost savings, and improved safety. By adopting predictive maintenance strategies, you can enhance the efficiency and longevity of your machinery, leading to better overall performance.
Every device has a point of failure. What does that mean? Well, a new device fresh from the manufacturer is healthy and problem-free. However, due to wear and tear as the device ages, its health slowly deteriorates until it eventually fails. At this point, you need to perform maintenance to restore the device to a healthy condition.
Maintaining equipment and machinery is crucial for ensuring smooth operations and maximizing efficiency in any industrial setting. Understanding the three main types of maintenance—reactive, preventive, and predictive—is essential for developing an effective maintenance strategy. Among these, predictive maintenance services stand out as a revolutionary approach that offers significant advantages over traditional methods. This comprehensive guide explores the three main types of maintenance, with a particular emphasis on the transformative impact of predictive maintenance services.
Reactive maintenance, also known as breakdown or corrective maintenance, is performed after equipment has failed. This type of maintenance is essentially a response to a problem rather than a proactive approach to preventing it.
Key Characteristics:
While reactive maintenance is often seen as a last resort, it is sometimes necessary for addressing immediate issues. However, relying solely on reactive maintenance can result in higher long-term costs and increased operational risks.
Preventive maintenance involves performing regular, scheduled maintenance tasks to prevent equipment failures before they occur. This approach is based on time intervals or usage metrics, aiming to keep equipment in optimal condition.
Key Characteristics:
Preventive maintenance is a proactive strategy that helps maintain equipment reliability. However, it may not always be the most cost-effective approach, as it does not consider the actual condition of the equipment.
Predictive maintenance services represent the pinnacle of modern maintenance strategies, leveraging advanced technology to anticipate equipment failures before they occur. This approach is based on real-time data analysis and condition monitoring, making it a highly effective and efficient method for managing equipment health.
Key Characteristics:
Benefits of Predictive Maintenance Services:
Each type of maintenance—reactive, preventive, and predictive—has its advantages and drawbacks. Here’s a quick comparison:
Understanding the three main types of maintenance—reactive, preventive, and predictive—is essential for developing an effective maintenance strategy. Predictive maintenance services, in particular, represent a significant advancement in maintenance practices, offering unparalleled benefits in terms of cost savings, efficiency, and equipment longevity.
Reactive Maintenance:
With reactive maintenance, you wait until a device breaks down before performing maintenance. For example, let’s say you have a microwave oven at home. You use it for a few years until it fails and won’t turn on anymore. In this case, you either repair the microwave or buy a new one. This process might take a couple of days, during which you can’t use your microwave. While this may not be a big deal at home, in a big industrial enterprise like an oil refinery, the consequences can be significant. For example, if a pressure transmitter fails and you wait to perform maintenance, the financial losses could be substantial due to interruptions in the manufacturing process. Thus, while reactive maintenance might work for a microwave, it is not ideal for industrial processes.
Preventive Maintenance:
The solution is preventive maintenance. With preventive maintenance, you perform maintenance before the device reaches the point of failure. For example, you can regularly check a pressure transmitter to ensure it doesn’t fail unexpectedly. However, this approach is not very cost-effective. Performing maintenance early wastes the remaining usable life of the device. This is where predictive maintenance comes in.
Predictive Maintenance:
As the name suggests, predictive maintenance involves predicting when a device will fail and scheduling maintenance just before that point. This minimizes downtime and maximizes the device’s lifetime. Predictive maintenance allows you to solve the downtime issue without wasting usable device life. It’s like cheating: you know when the device might fail, so you wait and then perform maintenance right before it happens.
In the realm of modern maintenance strategies, predictive maintenance services stand out for their ability to proactively manage equipment and machinery. By predicting potential failures before they occur, predictive maintenance services revolutionize how industries approach equipment upkeep. This article delves into the intricacies of how predictive maintenance works, exploring the key components and benefits of predictive maintenance services.
Predictive maintenance services involve monitoring equipment condition using advanced technologies to predict when maintenance should be performed. Unlike traditional maintenance methods, which can be reactive or scheduled, predictive maintenance is driven by data and analytics. This approach aims to anticipate problems before they lead to equipment failure, optimizing maintenance efforts and minimizing downtime.
Understanding how predictive maintenance services work involves examining several key components:
Data Collection: Predictive maintenance services begin with the collection of real-time data from equipment. This data is gathered through various sensors and IoT (Internet of Things) devices that monitor parameters such as temperature, vibration, pressure, and acoustic emissions. Accurate data collection is crucial for the effectiveness of predictive maintenance.
Condition Monitoring: The collected data is continuously monitored to assess the equipment’s health. Predictive maintenance services utilize sophisticated monitoring systems to track changes in performance and detect anomalies. By analyzing these conditions, maintenance teams can identify signs of potential issues before they escalate.
Data Analysis: Advanced analytics and machine learning algorithms play a critical role in predictive maintenance services. The data collected from equipment is analyzed to identify patterns and trends that indicate potential failures. Predictive models use historical data and current conditions to forecast when maintenance will be necessary.
Predictive Modeling: Predictive maintenance services employ predictive models to estimate the remaining useful life (RUL) of equipment components. These models take into account various factors, including operating conditions and historical performance, to predict when a part is likely to fail. This allows maintenance teams to plan interventions precisely.
Maintenance Planning: Based on the predictions and insights generated from the analysis, maintenance tasks are scheduled and planned. Predictive maintenance services enable precise scheduling of maintenance activities, ensuring that interventions are performed at the optimal time to prevent unexpected failures.
Feedback Loop: A crucial aspect of predictive maintenance services is the continuous feedback loop. After maintenance is performed, data is collected again to assess the effectiveness of the intervention. This feedback helps refine predictive models and improve the accuracy of future predictions.
The implementation of predictive maintenance services offers several significant advantages:
Reduced Downtime: By predicting potential failures and addressing issues before they cause significant problems, predictive maintenance services minimize unplanned downtime. This leads to uninterrupted operations and increased productivity.
Cost Savings: Predictive maintenance services help reduce maintenance costs by preventing major breakdowns and avoiding unnecessary maintenance tasks. By focusing on actual equipment conditions, organizations can save on repair costs and extend the lifespan of their assets.
Enhanced Equipment Longevity: Regular monitoring and timely maintenance interventions contribute to the extended lifespan of equipment. Predictive maintenance services ensure that machinery operates optimally, reducing wear and tear and preventing premature failures.
Improved Safety: Early detection of potential issues through predictive maintenance services enhances workplace safety. By addressing problems before they lead to equipment malfunctions, organizations can reduce the risk of accidents and create a safer working environment.
Increased Efficiency: Predictive maintenance services optimize maintenance scheduling and resource allocation. This results in more efficient operations and better overall performance, as maintenance activities are performed precisely when needed.
To effectively implement predictive maintenance services, consider the following steps:
Assess Equipment Needs: Identify which equipment and machinery will benefit most from predictive maintenance services. Focus on critical assets that have a significant impact on operations.
Select the Right Tools: Choose appropriate sensors, monitoring systems, and analytics tools that align with your equipment and operational requirements. Ensure compatibility with existing systems.
Train Your Team: Provide training for maintenance personnel to effectively use predictive maintenance tools and interpret data. Skilled teams are essential for maximizing the benefits of predictive maintenance services.
Monitor and Adjust: Continuously monitor the performance of predictive maintenance systems and make adjustments as needed. Regularly review data and predictions to refine maintenance strategies.
Evaluate Results: Assess the impact of predictive maintenance services on equipment performance, cost savings, and overall efficiency. Use this evaluation to inform future maintenance decisions and improvements.
Predictive maintenance services represent a significant advancement in maintenance strategies, offering a proactive approach to equipment management. By leveraging real-time data, advanced analytics, and predictive modeling, these services enable organizations to anticipate issues, reduce downtime, and optimize maintenance efforts.
Predictive maintenance uses data collected from similar devices in the past. By analyzing this data, we can predict when a similar device will fail. This process, often referred to as AI or Artificial Intelligence, involves analyzing historical and current data to make “intelligent” decisions for the future. One of these decisions is predicting device failure and scheduling maintenance accordingly.
At the time of posting this video, we’re still in the early phases of predictive maintenance. It may not yet work as accurately as it sounds on paper and can be expensive to implement. However, with ongoing advancements, the industry is gradually transitioning from preventive to predictive maintenance. This transition might take a few years or even decades.
A Thought Experiment:
As I was working on this video, I had some interesting thoughts. What if we could apply predictive maintenance to human body organs? What if we could replace a heart before it fails? Could we then live forever? Consider how the world would change if we could live indefinitely. Projects like Elon Musk’s SpaceX plan for making humans multi-planetary align with the idea of significantly extending human life. Let me know in the comments below what you think of this.
There are three types of maintenance: reactive, preventive, and predictive. Reactive maintenance involves fixing the device after it fails, causing production line stoppages and financial losses. Preventive maintenance involves regular checks and maintenance before failure, but it wastes usable device life. Predictive maintenance predicts when a device will fail and schedules maintenance just before that, minimizing downtime and maximizing the device’s lifetime.
Predictive maintenance services have revolutionized how organizations manage and maintain their equipment. By leveraging advanced technologies and data-driven insights, predictive maintenance helps predict potential failures before they occur. This proactive approach not only minimizes downtime but also enhances overall operational efficiency. In this article, we’ll explore the different types of predictive maintenance, providing a comprehensive guide to understanding the various methods and their benefits.
Predictive maintenance services involve using real-time data and advanced analytics to forecast equipment failures. Unlike traditional maintenance methods, which may be either reactive or scheduled, predictive maintenance focuses on assessing the condition of equipment to predict when maintenance is needed. This approach helps organizations perform maintenance activities just in time to prevent issues, optimizing both costs and performance.
There are several types of predictive maintenance services, each utilizing different techniques and technologies to monitor equipment health. Here’s an overview of the primary types:
Condition-Based Monitoring
Condition-based monitoring is a type of predictive maintenance that involves continuously monitoring the condition of equipment using various sensors. These sensors measure parameters such as temperature, vibration, and pressure. The data collected is analyzed to determine if any deviations from normal operating conditions indicate potential issues.
Key Features:
Benefits of Condition-Based Monitoring:
Vibration Analysis
Vibration analysis is a specialized form of predictive maintenance that focuses on monitoring and analyzing vibrations produced by equipment. Abnormal vibrations can indicate various issues such as imbalances, misalignments, or wear. By analyzing vibration patterns, maintenance teams can diagnose problems and plan interventions accordingly.
Key Features:
Benefits of Vibration Analysis:
Thermography
Thermography, or infrared thermography, is a predictive maintenance technique that uses thermal imaging to detect temperature variations in equipment. By analyzing thermal images, maintenance teams can identify hot spots, overheating components, and other temperature-related issues.
Key Features:
Benefits of Thermography:
Oil Analysis
Oil analysis involves testing the lubricating oil used in machinery to assess its condition and detect contaminants. By analyzing the properties of the oil, such as viscosity, acidity, and particle count, predictive maintenance services can identify potential problems such as wear, contamination, or lubricant degradation.
Key Features:
Benefits of Oil Analysis:
Acoustic Emission Monitoring
Acoustic emission monitoring involves listening to the sounds produced by equipment to detect abnormal noise patterns. This technique is useful for identifying issues such as leaks, cracks, and friction. By analyzing acoustic emissions, predictive maintenance services can diagnose problems before they lead to equipment failure.
Key Features:
Benefits of Acoustic Emission Monitoring:
To effectively implement predictive maintenance services, organizations should follow these steps:
Understanding the different types of predictive maintenance services is essential for optimizing equipment management and enhancing operational efficiency. From condition-based monitoring and vibration analysis to thermography, oil analysis, and acoustic emission monitoring, each method offers unique benefits for predicting potential failures and preventing downtime.
Like before, there could be a village that the person who senses it means like disgusting vibration is happening too much, so sometimes we use it like we record different types of meetings of the union. But it’s not like there’s too much vibration. Okay, so it will be known that the machine is vibrating too much, it means there’s a problem in it. Okay, in cases like this, if it seems that the machine is going to fail, then we try to find it and then try to remove that cord. So, this was basically what we do in product maintenance.
What we need to do next is edit it, okay, and then edit it so that it’s okay and to eliminate what causes it, so that it doesn’t happen again. What we’ve done so far is being used now, which is happening in the industry, and soon, the use of human sciences and other sensitive instruments. Workers’ attitudes increase. Subscribe, so what to do next is to guess, we’ll learn to use many instruments. Okay, like before, there could be a village that the person who senses it means like disgusting vibration is happening too much, so sometimes we use it like we record different types of meetings of the union. But it’s not like there’s too much vibration. Okay, so it will be known that the machine is vibrating too much, it means there’s a problem in it. Okay, in cases like this, if it seems that the machine is going to fail, then we try to find it and then try to remove that cord. So, this was basically what we do in product maintenance.
Okay, as before, I’m explaining to you very easily, it will be remembered very easily, so see the advantages. Understand the operation of nearly all the increasing components of maintenance. This is the same thing in response, no maintenance, and yes. So, the operational life of your component will increase. The machine will operate more for a long time, giving you more degrees. Equipment and process time is also coming to you, equipment time is going on because you’re letting fear be in it. Why are you letting it be? Because if there’s a problem, you’re trying to remove it first. Third, decrease in parts and labor costs. The part cost is decreasing, how is it decreasing? The part cost is being reduced. That’s how, for example, as I was saying, if there’s a rotating part, there’s a normal salt generating in it, it means there’s no sound. The sound is not coming, like from the motor, it’s coming anytime, so I feel like something is wrong with the motor.
Okay, now it’s rotating normally, the second one has become wire, if only what is needed is getting hot, it means something is shifting in the hotel or equipment and it can go bad later, so what will we do? At that time, we will stop the machine and try to find out what its problem is, alright, then we’ll stop the machine and try to find its problem, alright, next tagged interactive sentence, equipment condition, arm result periodic and on a continuous basis, and this enables maintenance men to make a timely action. Alert equipment adjustment, repair, and what do we do in the president? Either we are doing for periods or continue, sometimes randomly can also add that the machine is a part if it’s about to go bad, we already know it, so we’ll also change it in it, and we’ll do some work, but it’s failed, so we’ll do it first. So we’ll save a lot of time and avoid losses that occur due to breakdowns.
Next is predictive maintenance, tender service, live open equipment without fear of failure, this point has become completely clear to you, because when you’re cutting the point of maintenance before the breakdown, and you’re removing it, then your equipment’s life is going to increase, okay, so it’s obvious that you keep removing it by
paying attention to its name and eliminating the deficiency you find in it, so your equipment’s life will increase. Alright, moving forward, let’s take advantage of it. Advantage is a small topic, note it, it’s very easy. I’m explaining it to you very easily, so it will be remembered very easily, so see the advantages. We’ll add advantages, like nearly all increasing components of maintenance. Understand the operation of nearly all increasing components of maintenance. This is the same thing in response, no maintenance, and yes. So, the operational life of your component will increase. The machine will operate more for a long time, giving you more degrees. Equipment and process time is also coming to you, equipment time is going on because you’re letting fear be in it. Why are you letting it be? Because if there’s a problem, you’re trying to remove it first. Third, decrease in parts and labor costs. The part cost is decreasing, how is it decreasing? The part cost is being reduced.
That’s how, for example, as I was saying, if there’s a rotating part, there’s a normal salt generating in it, it means there’s no sound. The sound is not coming, like from the motor, it’s coming anytime, so I feel like something is wrong with the motor. Okay, now it’s rotating normally, the second one has become wire, if only what is needed is getting hot, it means something is shifting in the hotel or equipment and it can go bad later, so what will we do? At that time, we will stop the machine and try to find out what its problem is, alright, then we’ll stop the machine and try to find its problem, alright, next tagged interactive sentence, equipment condition, arm result periodic and on a continuous basis, and this enables maintenance men to make a timely action. Alert equipment adjustment, repair, and what do we do in the president? Either we are doing for periods or continue, sometimes randomly can also add that the machine is a part if it’s about to go bad, we already know it, so we’ll also change it in it, and we’ll do some work, but it’s failed, so we’ll do it first. So we’ll save a lot of time and avoid losses that occur due to breakdowns.
Next is predictive maintenance, tender service, live open equipment without fear of failure, this point has become completely clear to you, because when you’re cutting the point of maintenance before the breakdown, and you’re removing it, then your equipment’s life is going to increase, okay, so it’s obvious that you keep removing it by paying attention to its name and eliminating the deficiency you find in it, so your equipment’s life will increase. Alright, moving forward, let’s take advantage of it. Advantage is a small topic, note it, it’s very easy. I’m explaining it to you very easily, so it will be remembered very easily, so see the advantages. We’ll add advantages, like nearly all increasing components of maintenance. Understand the operation of nearly all increasing components of maintenance. This is the same thing in response, no maintenance, and yes. So, the operational life of your component will increase. The machine will operate more for a long time, giving you more degrees. Equipment and process time is also coming to you, equipment time is going on because you’re letting fear be in it. Why are you letting it be? Because if there’s a problem, you’re trying to remove it first. Third, decrease in parts and labor costs.
The part cost is decreasing, how is it decreasing? The part cost is being reduced. That’s how, for example, as I was saying, if there’s a rotating part, there’s a normal salt generating in it, it means there’s no sound. The sound is not coming, like from the motor, it’s coming anytime, so I feel like something is wrong with the motor. Okay, now it’s rotating normally, the second one has become wire, if only what is needed is getting hot, it means something is shifting in the hotel or equipment and it can go bad later, so what will we do? At that time, we will stop the machine and try to find out what its problem is, alright, then we’ll stop the machine and try to find its problem, alright, next tagged interactive sentence, equipment condition, arm result periodic and on a continuous basis, and this enables maintenance men to make a timely action. Alert equipment adjustment, repair, and what do we do in the president? Either we are doing for periods or continue, sometimes randomly can also add that the machine is a part if it’s about to go bad, we already know it, so we’ll also change it in it, and we’ll do some work, but it’s failed, so we’ll do it first. So we’ll save a lot of time and avoid losses that occur due to breakdowns.
With so much money, the biggest advantage of the village is this. To run in this way, you need technical requirements, youth, and internet connectivity for monitoring, as I mentioned. The equipment required is fine, such as the pressure gauge for the train’s bogie or a vibrating analyzer. To install all these, you need skilled individuals. In maintenance, you need experienced personnel. When they are off duty, the obvious thing is that their cost will be less. If you don’t hire them, your salary will increase. Okay, the next point is about increased investment in this area. This point is related to understanding its significance if you have to engage in trading. You’ll have to increase investment in trading, which will, in turn, lead to its advantages. However, there may be disadvantages in predictive maintenance.
In the world of equipment management and maintenance, predictive maintenance services have emerged as a game-changing approach. Predictive maintenance leverages real-time data and advanced analytics to forecast potential failures, making maintenance activities more efficient and effective. But what exactly makes predictive maintenance easy to implement and beneficial for organizations? This article explores the key factors that simplify predictive maintenance services, highlighting why they are a valuable addition to any maintenance strategy.
Predictive maintenance services involve monitoring equipment conditions using various technologies and analytics to predict when maintenance should be performed. Unlike traditional maintenance methods, which are often reactive or scheduled based on time intervals, predictive maintenance focuses on the actual state of the equipment. This proactive approach helps prevent unexpected failures and minimizes downtime.
Real-Time Data Collection
One of the most significant advantages of predictive maintenance services is the ability to collect real-time data from equipment. Modern sensors and IoT devices continuously monitor parameters such as temperature, vibration, and pressure. This real-time data collection simplifies the process of tracking equipment health and detecting anomalies early.
Benefits:
Advanced Analytics and Machine Learning
Predictive maintenance services leverage advanced analytics and machine learning algorithms to analyze collected data. These technologies process vast amounts of information to identify patterns and predict potential failures. The use of sophisticated analytics makes it easier to forecast maintenance needs accurately.
Benefits:
Integration with Existing Systems
Many predictive maintenance services are designed to integrate seamlessly with existing maintenance management systems and enterprise resource planning (ERP) platforms. This integration makes it easier to incorporate predictive maintenance into current workflows and ensures a smooth transition from traditional maintenance methods.
Benefits:
User-Friendly Dashboards and Interfaces
Predictive maintenance services often come with user-friendly dashboards and interfaces that simplify data visualization and interpretation. These tools allow maintenance teams to easily monitor equipment conditions, view predictions, and make informed decisions based on clear and actionable insights.
Benefits:
Scalability and Flexibility
Predictive maintenance services are designed to be scalable and flexible, making them suitable for organizations of various sizes and industries. Whether managing a few assets or an extensive network of equipment, predictive maintenance solutions can be tailored to meet specific needs and grow with the organization.
Benefits:
Proactive Maintenance Planning
Predictive maintenance services enable proactive maintenance planning by providing insights into when and what type of maintenance is needed. This proactive approach ensures that maintenance activities are scheduled based on equipment condition rather than arbitrary time intervals.
Benefits:
Enhanced Support and Training
Many predictive maintenance service providers offer comprehensive support and training to help organizations implement and utilize their solutions effectively. This support makes it easier for teams to adopt predictive maintenance practices and maximize the benefits of the technology.
Benefits:
Predictive maintenance services offer a modern, data-driven approach to equipment management that simplifies maintenance processes and enhances efficiency. Key factors such as real-time data collection, advanced analytics, seamless integration, user-friendly interfaces, scalability, proactive planning, and robust support contribute to making predictive maintenance easy to implement and highly effective.
Equipment manufacturers in numerous industries, such as medical devices, high-volume pumps, packaging equipment, data centers, and more, have implemented the Industrial Internet of Things (IIoT) in production and achieved initial value. But why stop short of realizing the full potential of IIoT connectivity?
With predictive maintenance and service, you can maximize the use of your data to increase your customers’ uptime. When you use your connected data stream to set alarms and alerts based on predetermined points, you are already making predictions. These predictions are based on your knowledge of your equipment’s performance limits, test data, and field experience. Now, unlock the greater potential of your data to deliver business benefits.
Look to predictions that better target problems before they arise, bringing significant benefits to your organization and customers. Predictive service models help you save costs by reducing truck rolls, while customers can gain millions in increased uptime, productivity, and ROI as equipment is fixed before it breaks.
Because you have the data at your disposal to potentially save your customers millions of dollars in reduced downtime, customer satisfaction will increase. This leads to higher renewal rates, lower churn, and better net promoter scores. These substantial benefits stem from predicting not only based on existing knowledge but also on what you continue to learn.
With predictive tools, you can construct statistical models based on data gathered over time. Machine learning enables these tools to automatically update and improve models as you learn more, without requiring hands-on time from anyone on your team or a data scientist. Experts at PTC are ready and available to ensure that all your predictive maintenance tools are easily incorporated into your business processes.
You already make predictions based on what you know. Leverage the full potential of IIoT by using what you learn, and reap the documented ROI, increased uptime, and greater customer satisfaction that your data provides.
Artificial Intelligence (AI) has become a transformative force in various industries, and predictive maintenance services are no exception. AI-based predictive maintenance leverages cutting-edge technologies to forecast equipment failures, optimize maintenance schedules, and enhance operational efficiency. This article explores how AI-based predictive maintenance is revolutionizing predictive maintenance services and why it is a game-changer for modern industries.
AI-based predictive maintenance integrates artificial intelligence with predictive maintenance services to analyze data, detect anomalies, and predict equipment failures with unprecedented accuracy. Unlike traditional methods that rely on fixed schedules or reactive approaches, AI-based solutions use machine learning algorithms to process vast amounts of data and provide actionable insights for proactive maintenance.
Advanced Data Analytics
AI-based predictive maintenance services utilize advanced data analytics to process and interpret complex datasets. Machine learning algorithms analyze real-time data from sensors and IoT devices to identify patterns and trends that may indicate potential equipment failures. This enhanced data analysis provides deeper insights and more accurate predictions than traditional methods.
Benefits:
Anomaly Detection
AI-based predictive maintenance services excel in anomaly detection by continuously monitoring equipment performance and identifying deviations from normal behavior. Machine learning models are trained to recognize patterns associated with failures, allowing for early detection of issues before they escalate into major problems.
Benefits:
Predictive Modeling
Predictive modeling is a core component of AI-based predictive maintenance services. AI algorithms create models based on historical data and current equipment conditions to predict the remaining useful life (RUL) of components. These models help maintenance teams schedule interventions at the optimal time, ensuring that maintenance activities are performed when necessary.
Benefits:
Automated Maintenance Recommendations
AI-based predictive maintenance services automate the generation of maintenance recommendations based on data analysis and predictive modeling. These recommendations provide actionable insights on when and what type of maintenance is required, streamlining the maintenance planning process and reducing the burden on maintenance teams.
Benefits:
Integration with Existing Systems
AI-based predictive maintenance services are designed to integrate seamlessly with existing maintenance management systems and enterprise resource planning (ERP) platforms. This integration ensures that predictive maintenance insights are easily accessible and actionable within the organization’s existing workflows.
Benefits:
Scalability and Adaptability
AI-based predictive maintenance services are scalable and adaptable, making them suitable for organizations of various sizes and industries. Whether managing a few assets or an extensive network of equipment, AI-based solutions can be tailored to meet specific needs and scale with the organization’s growth.
Benefits:
Continuous Learning and Improvement
One of the key advantages of AI-based predictive maintenance services is their ability to continuously learn and improve over time. Machine learning algorithms refine their predictions and recommendations as more data is collected, leading to increasingly accurate forecasts and better maintenance outcomes.
Benefits:
To effectively implement AI-based predictive maintenance services, organizations should consider the following steps:
AI-based predictive maintenance services represent a significant advancement in equipment management, offering enhanced accuracy, efficiency, and reliability. By leveraging advanced data analytics, anomaly detection, predictive modeling, and automation, AI-based solutions revolutionize traditional maintenance practices.
Can you predict when you will have a system or machine outage in the future? Imagine how much time, money, and headaches this could save you. Aditya Baru, the Senior Product Manager at MathWorks, shares his experience on how artificial intelligence (AI) can help prevent not just outages but also headaches.
Some examples where AI-based predictive maintenance works best
Is my machine behaving abnormally? This is anomaly detection.
Is there a fault in my system, and what’s the root cause? This is fault classification or root cause isolation.
When is my system going to fail? This is the concept of remaining useful life estimation.
For each of these, you can use image data and sensor data in different ways. For example, a major oil and gas company uses MATLAB with sensor data to predict when their trucks might fail. They collect sensor data from their equipment, analyze it using signal processing techniques, and then run the features from these techniques through machine learning models that can detect the presence of faults in their trucks.
When it comes to image-based data, a major aircraft manufacturer uses MathWorks tools for deep learning. They take images of aircraft components and run them through deep neural networks to detect defects or faults. This method is much simpler than manually extracting features from images and figuring out what’s going on in them. The ability to use AI, machine learning models, and deep learning models has made it much easier to solve these problems.
Predictive maintenance services have emerged as a transformative approach to equipment management, leveraging real-time data and advanced analytics to predict and prevent failures. However, despite their significant benefits, there are several challenges in the marketplace related to the implementation and adoption of predictive maintenance services. Understanding these challenges is crucial for organizations looking to optimize their maintenance strategies and make the most of predictive maintenance solutions.
Data Quality and Integration
One of the primary challenges associated with predictive maintenance services is ensuring high-quality data collection and integration. Effective predictive maintenance relies on accurate and comprehensive data from various sensors and IoT devices. Poor data quality or integration issues can lead to incorrect predictions and reduced reliability of maintenance forecasts.
Challenges:
Solutions:
High Initial Costs
The implementation of predictive maintenance services often involves significant upfront costs. These costs include the acquisition of advanced sensors, data analytics tools, and AI technologies. For many organizations, particularly small and medium-sized enterprises, these initial investments can be a substantial barrier to adoption.
Challenges:
Solutions:
Complexity of Implementation
Implementing predictive maintenance services can be complex due to the need for specialized knowledge and skills. The integration of AI, machine learning, and data analytics into existing maintenance systems requires technical expertise and can be challenging for organizations without prior experience.
Challenges:
Solutions:
Data Security and Privacy
As predictive maintenance services rely on the collection and analysis of large volumes of data, data security and privacy become critical concerns. Protecting sensitive information from cyber threats and ensuring compliance with data privacy regulations are essential for maintaining the integrity of predictive maintenance systems.
Challenges:
Solutions:
Maintenance of Predictive Systems
Once implemented, predictive maintenance systems require ongoing maintenance and updates. Ensuring that predictive models remain accurate and effective over time involves continuous data monitoring, model retraining, and system maintenance.
Challenges:
Solutions:
Lack of Standardization
The predictive maintenance services market lacks standardization, which can lead to compatibility issues and difficulties in comparing different solutions. The absence of industry-wide standards makes it challenging for organizations to evaluate and select the most suitable predictive maintenance tools.
Challenges:
Solutions:
While predictive maintenance services offer significant advantages for equipment management and operational efficiency, several challenges in the marketplace must be addressed. From data quality and integration to high initial costs and implementation complexity, organizations need to navigate these obstacles to successfully adopt and benefit from predictive maintenance solutions.
To understand the difficulties associated with predictive maintenance, it’s important to realize the process involved in building a predictive maintenance solution. Engineers need to:
Extract features from that data or reduce the data set.
Build a predictive model to detect failures or predict when a failure might occur.
Integrate this predictive model with their production environment, whether it’s an IT system, an OT system, or anything else.
Each stage presents its own challenges. For data acquisition, it’s hard to get the right kind of data. Failure data is especially hard to obtain because engineering companies work to prevent equipment failures. Even if data is acquired, it needs to be reduced to a manageable size. Standard industrial equipment today can generate gigabytes to terabytes of data daily, making it difficult for engineers and data scientists to process all this data cost-effectively and efficiently. Feature extraction and data reduction become crucial here.
Assuming you overcome the hurdle of data extraction, training a machine learning model is the next step. While data scientists are familiar with machine learning models, engineers often are not. They need to determine which machine learning model to use for the best predictive maintenance algorithm. Finally, integrating the predictive maintenance algorithm into the production environment is essential. Without successful integration, you cannot achieve the benefits of a predictive maintenance solution. The variations in infrastructure, whether cloud-based, on-premises, or hybrid systems, and the number of edge devices can complicate this integration process.
Predictive maintenance services have become a cornerstone for optimizing equipment management and improving operational efficiency. However, organizations often face several challenges when implementing these advanced solutions. To effectively address these challenges, it’s crucial to understand the necessary skills and tools required for successful deployment and management of predictive maintenance services. This article explores how organizations can overcome common challenges, and the key skills and tools needed for effective predictive maintenance.
Overcoming Data Quality and Integration Issues
Challenges:
Solutions:
Skills and Tools Required:
Managing High Initial Costs
Challenges:
Solutions:
Skills and Tools Required:
Navigating Implementation Complexity
Challenges:
Solutions:
Skills and Tools Required:
Ensuring Data Security and Privacy
Challenges:
Solutions:
Skills and Tools Required:
Maintaining Predictive Systems
Challenges:
Solutions:
Skills and Tools Required:
Addressing the Lack of Standardization
Challenges:
Solutions:
Skills and Tools Required:
While predictive maintenance services offer significant advantages, organizations must address various challenges to effectively implement and manage these solutions. By investing in the right skills and tools, organizations can overcome obstacles related to data quality, costs, implementation complexity, security, system maintenance, and standardization.
At the heart of a predictive maintenance solution should be an engineer who brings domain expertise to the problem. For example, engineers can help obtain the right kind of failure data by using models built during the design stages to generate synthetic failure data by injecting failure modes and faults. This makes the process of gathering failure data safer and more cost-efficient.
Once failure data is obtained, extracting features from it is the next step. Engineers’ domain expertise is crucial here. They understand what the machine is supposed to do and how the machine’s data might look if it’s approaching failure modes or fault conditions. This knowledge needs to be incorporated into the predictive maintenance solution.
The aspect of training a machine learning model can be addressed using tools from companies like MathWorks or by collaborating with data scientists. This allows experimentation with different machine learning and deep learning models to determine what works best for the application.
Finally, deploying the predictive maintenance algorithm involves working with IT and OT organizations within the company. These teams understand the infrastructure and how the algorithm needs to integrate to achieve the desired benefits. Whether it’s integrating with edge devices, ERP systems, or data historians, collaboration ensures successful integration into the production system.
We will focus on industrial applications that leverage cyber-physical systems and Internet of Things (IoT) functionalities, as well as some of the presented digital enablers of the Fourth Industrial Revolution. One of the main business drivers of the Fourth Industrial Revolution has been the transition from mass production to mass customization.
The Third Industrial Revolution was characterized by the emergence of the production line that could produce large amounts of the same products in a cost-effective manner. This gave rise to mass production and made-to-stock production models. The Fourth Industrial Revolution enables more flexible production lines that can produce multiple variations of a product as part of a mass customization paradigm. Flexible production lines lower the cost of producing customized products. This is because sensors and IoT devices enable the production line to automatically identify individual orders and production recipes, and accordingly configure the line to produce customized products. For instance, by reading a tag or a QR code on a production order, the required production configuration can be identified. Then, a flexible line can configure industrial robots to produce diversified products in line with the identified production configuration.
Cyber-physical systems and IoT devices enable the creation of modular production lines that are very flexible. Sensors, automation devices, networking devices, and software modules can act as Lego-like building blocks of a flexible production line. Proper modules and interfaces between them can then be selected to support customized production configurations and propel mass customization paradigms like made-to-order.
Another technology that boosts the flexibility of production lines is additive manufacturing or 3D printing technology. 3D printers enable the printing of specific products or parts, which can accelerate the production process. Most importantly, 3D printing enables a novel manufacturing paradigm, namely manufacturing as a service (MaaS). MaaS is an on-demand production paradigm where entire products or their parts are ordered through a cloud-based infrastructure and executed based on 3D printers. In this direction, there is a need for sharing a digital model of the product through a secure and trusted manufacturing network. The model can then enable the activation of the additive manufacturing process. Additive manufacturing can be used as an element of a flexible production line. Specifically, it is possible to print a part or component of a product, increasing the flexibility and speed of the production process.
One of the most popular applications of the Fourth Industrial Revolution is predictive maintenance of physical assets in industrial environments. Predictive maintenance is the next evolutionary step in the management of assets in industrial environments. Once upon a time, assets like industrial machinery were maintained based on a reactive approach. This meant that assets were repaired, maintained, or replaced when they failed. This maximized the use of individual assets but resulted in catastrophic unplanned downtimes that stopped production operations. Hence, it was a problematic approach that led industrial enterprises to transition to preventive maintenance approaches.
In the scope of a preventive maintenance approach, unplanned downtime is avoided as assets are maintained before the end of their life. However, this results in underutilization of the assets, as preventive maintenance approaches do not fully exploit their useful life. To alleviate this limitation, enterprises are seeking ways to predict the remaining useful life of assets based on their condition. This is typically a very difficult problem, which can be solved by leveraging big data and artificial intelligence (AI) technology. To assess the actual condition of the asset, industrial organizations can collect and combine information from a variety of sensors, including vibration sensors, acoustic sensors, ultrasonic sensors, thermal imaging sensors, temperature sensors, and more. This information can be combined with other sources such as oil analysis. Accordingly, deep learning and AI algorithms can be used to predict and anticipate the exact time when the equipment will fail. This leads to optimal utilization of the assets while avoiding downtime. The economic benefits of such an approach can be huge, especially for very expensive assets like machines that cost several millions of euros. Increasing the utilization of such assets can have a positive impact on an enterprise’s economic output and bottom line.
Predictive maintenance is a very popular application of the Fourth Industrial Revolution. Its popularity is due to the fact that it can be used in virtually all industrial sectors that manage physical assets, including manufacturing, oil and gas, mining, smart buildings, and public infrastructure projects like smart city initiatives.
Industry 4.0 technologies also enable the development and use of powerful applications based on the concept of a digital twin. A digital twin implements a faithful digital representation of physical assets and processes in the digital world. For instance, a digital twin can provide a representation of industrial machinery and its operations, or even an entire production line. A digital twin is constructed based on the collection and structuring of large amounts of digital information about the physical process by means of sensors and appropriate data models of the physical world. The implementation of physical processes in the digital world provides industrial organizations with a means of experimenting with physical processes without the pitfalls of trial and error in the physical world. This is because digital models can be easily changed and reverted back to a previous condition, which is not possible with physical objects. Consider, for example, a drawing in your PowerPoint program. You can easily change objects in the drawing until you find the proper configuration of the shapes. This cannot happen in the physical world with physical processes. For example, when you throw some drops of milk in a cup of coffee, it is almost impossible to undo this action and revert back to the previous condition. Hence, digital twins enable industrial organizations to design and execute simulations of physical phenomena prior to their actual implementation in the real world.
In this slide, you can see a digital twin for the simulation of a product sorting process across different bays of a conveyor belt. The digital twin enables the manufacturer to experiment with different sorting strategies and select the best one prior to implementing it on the manufacturing shop floor. Digital twins can be combined with metaverse and extended reality functionalities. These functionalities can enhance digital twin applications with realistic visualizations of physical processes, making trial and error experimentation more pragmatic. Metaverse simulations include, for example, what-if analysis about different production configurations or asset maintenance strategies.
The digital twin concept has its roots in manufacturing and other industrial sectors. It is extensively used to simulate production, maintenance, and quality control processes. In recent years, the concept has also been extended to other areas. As a prominent example, in the healthcare sector, digital twins are used to create and simulate patient-centric clinical processes such as disease treatment. To this end, a patient digital twin is constructed based on the integration of data from a variety of sources, including patient databases, medical images, laboratory examinations, real-world data about the patient, the patient’s family history, genomics information, and more. The creation of an integrated and accurate digital representation of the patient is a very powerful tool for clinicians and other healthcare professionals.
Beyond digital twins, Industry 4.0 applications can be used to optimize the operation of supply chains. Nowadays, it is possible to collect more supply chain data than ever before. For instance, it is possible to track and trace products based on sensors and automatic identification technologies. It is also possible to acquire information about the operational status of equipment and machinery. These pieces of information can be analyzed to provide accurate forecasts of supply, demand, and other operational parameters of the supply chain.
Overall, the Fourth Industrial Revolution enables many different applications that improve the competitiveness of industrial organizations based on improvements in their production operations and in their supply chains.
Facilio:
Here is a quick preview of what we’re going to do. There are three quick sections I wanted to touch upon: what Vasily provides, a new way to unify building data access, what it means to modern real estate companies, and flexible strategies that we can implement right now for building performance and tenant experience. By the end of these sessions, you will know more about how Vasily helps property owners optimize building performance and tenant experience.
This is a quick slide, and I’ll also show a couple of screens to touch upon some key aspects. So, what are some of the problems that CRE professionals face in today’s world? There is no scope to optimize operating costs, lack of visibility across buildings and portfolios, and it’s tedious to manage or scale up innovation, making it challenging to deliver value to property owners or tenants.
This is a three-tier architecture in terms of how Vasily delivers value. We start with a data collection layer, which can connect to legacy automation systems such as BMS, HVAC, elevators, and fire safety systems. We also have connectivity to various IoT sensors and devices, such as people counting or energy sensors, which feed into our operations cloud platform. It’s not just a data cloud platform; this is where we aggregate all building data in real-time. It has its own layer of analytics engines, reports, and dashboards, which feed into our integrated application experience. These experiences include tenant experience management, maintenance management, or a connected building suite.
This leads to what we call a hardware-agnostic operations cloud. We support any protocol that can connect to a BMS, such as BACnet, Modbus, OPC, and other protocols you can see on the screen. This takes us to the value proposition that Vasily delivers. It provides a complete operational picture of the entire platform, reduces carbon footprint by supporting sustainability initiatives, enhances tenant engagement and experience, increases asset value, and reduces operational costs.
Having said that, let me dive into a quick demo. It’s going to be a persona-driven demo showing how Vasily caters to different roles, such as an executive, a portfolio manager, or an operations supervisor. I’ll take everyone through how data flows between these different stakeholders on the platform. This will ensure they’re all connected and always staying in context, enabling them to make data-driven decisions in their everyday lives.
Let’s start with a quick view of how Vasily creates a portfolio-level digital replica of a building. As you can see, there are different properties and buildings, which gives a connected, aggregated data view. For example, if I click on a certain building, Vasily pulls data from every connected system, human factors, and external conditions. I could start my day as an executive by saying, “Okay, I’m looking into 110 Central Avenue. Right now, I have five work order tickets open, 20 alerts to be acknowledged, and 116 assets and equipment under this building. My total energy consumption is 81 megawatts per hour.”
Continuing as an executive, I will focus on three different aspects. If I’m the head of sustainability, I’ll look at my costs, overall consumption, and energy efficiency. If I want to quickly see my building’s cost performance, I can check my top five performing properties or bottom five performing properties. If I want to examine the energy usage intensity of various buildings, the colors will indicate whether energy consumption is high or low and if sustainability initiatives are needed.
If I have a problem with maintenance, I’ll talk to my portfolio manager. He logs in and sees from his supervisory dashboard the status of work orders and tickets. We have custom views to check each work order by department. I can view a complete plant maintenance calendar, a workforce calendar, and even set up customized workflows for different maintenance types, tenants, or buildings.
Jumping from here, I can check the entire asset health lifecycle. I get a complete picture of maintenance activities, live readings, performance, maintenance costs, and live graphics views of how all this data adds up. From an energy management perspective, I can see my top ten energy-consuming assets. I can implement energy conservation measures, figure out target savings, track energy consumed, and monitor the savings after the measures are implemented.
So, having lost some time, that’s a quick rapid-fire perspective of what Vasily offers. Please log on to www.vasily.com. You can request a detailed live demo and visit our “Get Started” section to explore more use cases. I know we have a couple of minutes left, Ash, so I can take any questions from here.
Great. For those with questions about Vasily, please click on the Q&A button at the bottom of your page. I see a question has come through: “What is the average time to connect buildings and acquire data from siloed vendor systems?”
That’s a good question. Typically, the perception is that it takes a lot of time, but it usually takes about three to four weeks, depending on the scale of the building system. It’s also influenced by how fast the customer teams can come on board and share access. Since it’s an IoT edge platform, I’d say it takes about three to four days to get started, and we can usually complete it in three to four weeks.
Another question: “How is ROI calculated?”
ROI is calculated based on several factors, such as energy consumption and energy bills. We use flexible diagnostics and ML-based algorithms to connect, optimize, and predict future energy consumption and asset downtime, ultimately determining the savings.
Upkeep, Fiix, Simple CMMS:
Our top pick for the best overall CMMS software is MaintainX. Trusted by industry leaders like Hilton Hotels, McDonald’s, Marriott, and Burger King, MaintainX excels in maintaining facilities, equipment, safety standards, and communication within maintenance departments. Its features include unlimited work orders, assets, locations, photos, and messages, a highly responsive mobile app, form creation templates, and collaborative workflows. MaintainX is tailored for manufacturing, industrial businesses, schools, country clubs, facilities management, and property management. Pricing starts at $16 per month for the Essential plan, making MaintainX our top recommendation.
Next up, Maintenance Care is our top choice for a free CMMS solution. With over 30,000 users managing 10+ million assets, Maintenance Care is perfect for businesses transitioning from Excel spreadsheets or paper records. The software offers work orders, asset tracking, parts and inventory management, preventative maintenance, document storage, wireless sensors, reporting, and integrations. Pricing starts at $1,100 per month billed annually, with a free forever plan for basic use and online work order management. Maintenance Care is trusted by property managers, small businesses, senior care centers, government organizations, hospitality businesses, and manufacturing companies.
For the best CMMS mobile app, UpKeep takes the lead. A mobile-first solution, UpKeep is ideal for technicians and agile maintenance teams on the go. With features like work orders, maintenance checklists, inventory management, and workflow automation, UpKeep is trusted by over 150,000 maintenance professionals worldwide. Pricing starts at $145 per user per month for the Starter plan, $175 for Professional, and custom pricing for Business Plus.
Limble CMMS stands out as the best choice for enterprise asset management (EAM), offering tools for detailed maintenance logs, real-time asset data, KPI tracking, and QR codes. Limble CMMS is suitable for organizations with a large number of assets. Plans start at $155 per user for the Starter package, with a 30-day free trial. Limble CMMS provides scalability and customization for various needs.
If you’re looking for extensive features, MicroMain is the way to go. Trusted by 10+ customers, including the US Army, Walmart, and John Deere, MicroMain offers a broad range of features such as events, metering triggers, KPI tracking, user role permissions, real-time reporting, and more. Pricing is based on admin and technician licenses, starting at $99 for admin and $45 for technicians. Contact the MicroMain sales team for a free quote and a 14-day free trial.
Hippo CMMS takes the spotlight as the best web-based CMMS. Though not the most popular, it’s chosen for its simplicity and ease of use. With features like work order management, equipment management, predictive maintenance, and vendor management, Hippo CMMS is suitable for those seeking a straightforward web-based solution. Pricing is not available online, but you can try Hippo CMMS free for 14 days.
eMaint is recognized as the best CMMS software for scalability. Trusted by over 50,000 users globally, eMaint offers robust tools for enterprise asset management, work order management, scheduling, and reporting. eMaint is adaptable as your business grows. Pricing starts at $133 per user per month with a three-user minimum for the entry-level plan. For advanced features and over three users, pricing jumps to $185 per user per month, with custom enterprise rates available.
Lastly, Fiix is the best choice for simple work orders. Core features include work order management, asset management, parts and supplies management, and reporting. With a free plan for up to three users and paid plans starting at $145 per user per month, Fiix is an affordable solution for both small and large teams.
Choosing the best CMMS software for your business involves considering factors like core and additional features, ease of use, business size, and pricing. Evaluate your specific needs against the features offered by these top solutions to find the perfect fit. Remember, there’s no one-size-fits-all solution, so make an informed decision based on your unique requirements.
IBM Maximo:
Organizations often struggle with tracking asset location and availability. However, it’s not just about assets; scheduling skilled technicians for service and repair orders, increasing compliance with environmental and health regulations, and meeting reporting requirements are also significant challenges organizations face.
Why use IBM Maximo to address these challenges? Maximo enables you to track and manage asset deployment, specifications, conditions, and calibration costs all in one system. Additionally, with the contracts module, you can manage vendor contracts comprehensively, including purchase, lease, rental, warranty, blanket contracts, and user-defined contracts. On the inventory side, Maximo helps meet maintenance demand accurately, ensuring the right parts are available at the right location when needed.
Delving deeper into Maximo, let’s explore the product portfolio. Starting with the core solution, we have Maximo EAM Core, available as an on-premise solution. On the other side, we have Maximo Enterprise Asset Management (EAM) powered by IBM Maximo, or as we call it, Maximo, which is a comprehensive solution. With this core, you can add industry-specific solutions, and IBM continues to develop new ones.
Maximo also offers add-on solutions, such as Maximo Scheduler, which allows you to graphically schedule and assign labor with the necessary materials for their work. Maximo Anywhere, a mobile solution, enables offline and online work, offering a unique functionality compared to other mobile solutions within Maximo, such as the work centers.
Integration is another strength of Maximo. It offers out-of-the-box integration with IBM Control Desk, providing a seamless combination of IBM Maximo and IBM Control Desk in one system. This integration supports IT Service Management (ITSM) with asset management, IoT-driven solutions like Maximo Monitor and Maximo Health for 24/7 asset health monitoring.
Next, let’s discuss IBM Maximo processes. Central to Maximo is the asset module, which includes asset management with meter readings, condition monitoring, failure hierarchy, and failure codes. This setup enables technicians to report failures effectively. Work management includes job plans, preventive maintenance, and automatic work order creation based on schedules or meter readings. Inventory management encompasses purchasing, automatic reordering, and planning and scheduling. Health and safety features include lockout/tagout procedures and safety plans to ensure a safe
working environment for technicians. Maximo also integrates smoothly with ERP systems like SAP or Microsoft Dynamics, thanks to its open integration framework.
Now, let’s dive deeper into specific processes, starting with asset management. Essential functionalities include total location and asset structure, work order history, and safety and hazard elimination within the safety module. The asset management module allows for condition monitoring, failure codes, and meter groups. Here’s a glimpse of the asset application, showcasing a list view where you can filter and search for assets. Detailed views include general information, location, meter groups, and attachments like pictures of the asset.
Next, let’s discuss work management. Starting with self-service, users can create service requests by classifying issues, describing them, and adding photos or videos. Supervisors can review these requests, create work orders, and apply job plans, which outline necessary tasks, labor, and materials. Preventive maintenance schedules can automatically generate work orders based on predefined intervals or meter readings. Maintenance technicians can use work centers to view and update their work orders, report work time, materials used, and failures encountered.
The supply chain process involves managing contracts, purchasing, and inventory. Maximo’s functionality supports receiving materials, inventory counts, and automatic reordering. The analytics module offers query-based reporting, custom reports, and Excel integration. Business analysts can use work centers to visualize work management data through KPIs and graphical representations.
To summarize, IBM Maximo EAM benefits various departments by reducing unplanned downtime, inventory costs, and extending asset life. It enhances asset reliability and minimizes asset failures, ensuring assets are operational and well-maintained. home
Moreover, Prodigifirm is more than just a platform – it’s a community. Join thousands of like-minded professionals from around the globe who are on the same journey towards personal and professional growth.
© 2024 Prodigifirm. All Rights Reserved.